Welcome to the webpage of the TAUDoS project
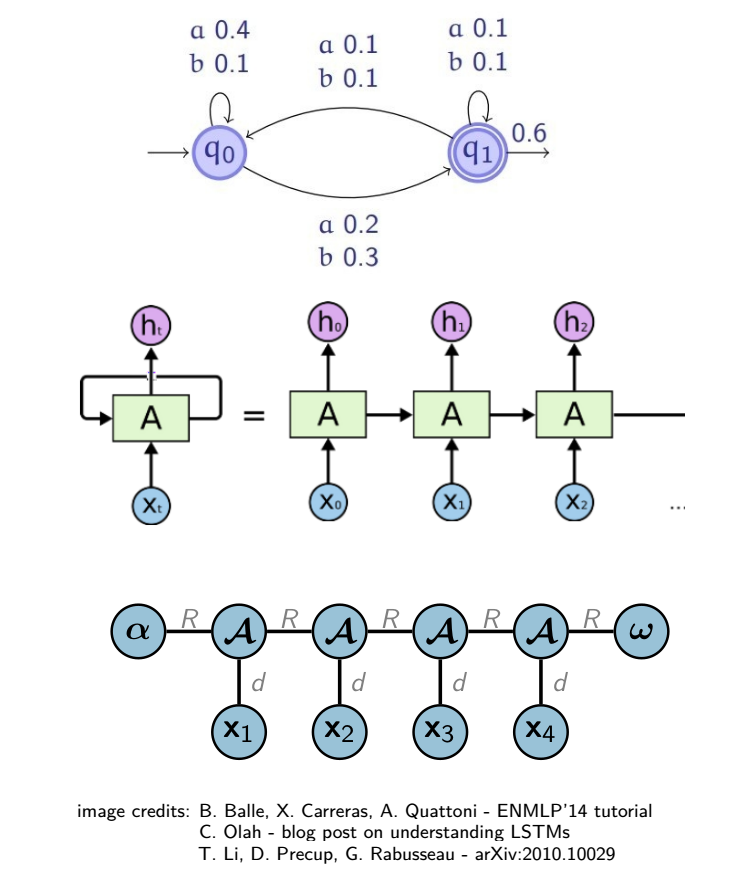
TAUDoS is a 4 years project (2021-2024) funded by the Agence Nationale de la Recherche via the PRCE program.
Its consortium regroups researchers of 5 differents labs:
- The Hubert Curien Laboratory of the Jean Monnet University
- The Laboratoire d'Informatique et Systèmes of the Aix-Marseille University
- The R&D center of the EURA NOVA firm
- The Département d'Informatique et de Recherche Opérationnelle of the Université de Montréal
- The Laboratoire des Sciences du Numérique de Nantes of the Nantes University
The ambition of our project is to provide a better understanding of the mechanisms that allow the amazing recent achievements of Machine Learning, and in particular of Deep Learning. This will be achieved by providing elements that allow a better scientific comprehension of the models, strengthening our experimental results by theoretical guarantees, incorporating components dedicated to interpretability within the models, and allowing trustful quantitative comparison between learned models.
The originality and the specificities of TAUDoS are due to three major characteristics:
- The focus on models for sequential data, such as Recurrent Neural Networks (RNN), while most works concentrate on feed-forward networks
- The will to analyse these models in the light of formal language theory
- The goal to target both rigorous theoretical analyses and empirical evidence related to interpretability.
More precisely, TAUDoS follows 4 interconnected scientific objectives. The first one is to provide theoretical studies both to characterize classes of learnable RNN in connection with formal languages classes and to design provable learning algorithms for them. The second one is to propose new knowledge distillation algorithms to extract simpler, more understandable models from deep RNN, either from the whole model or by identifying interesting subparts. The third idea is to design new learning strategies to favor interpretability of RNN in the training stage, either by incorporating structural elements in the architecture or by including in the objective function to be optimized additional terms dedicated to interpretability. Finally, the project aims at initiating the research path of learning metrics to compare RNN on a given task, in order to better understand and mine the topical zoo of RNN architectures.
While the core of the project is to bring better understanding of deep learning, TAUDoS will hopefully also enjoy potentially great side-effects : new initialisation procedures and new learning algorithms for RNN, models of equivalent quality to huge deep ones that require far less computation power, new interesting classes of formal languages to study in order to understand the capacities of RNN, new strategies for favouring interpretability, new metrics between models that can be clues for the transferability of interpretability, ...
In addition to the undeniable scientific impacts that the advancements of TAUDoS will have, the presence in the consortium of a R&D firm ensures its valorization.
Furthermore, TAUDoS comes within the scope of the open science movement: new benchmarks to help answer the hard question of the evaluation of interpretability will be freely available, and a user-friendly open source toolbox containing our algorithms will be developed.